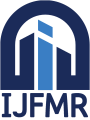
International Journal For Multidisciplinary Research
E-ISSN: 2582-2160
•
Impact Factor: 9.24
A Widely Indexed Open Access Peer Reviewed Multidisciplinary Bi-monthly Scholarly International Journal
Home
Research Paper
Submit Research Paper
Publication Guidelines
Publication Charges
Upload Documents
Track Status / Pay Fees / Download Publication Certi.
Editors & Reviewers
View All
Join as a Reviewer
Reviewer Referral Program
Get Membership Certificate
Current Issue
Publication Archive
Conference
Publishing Conf. with IJFMR
Upcoming Conference(s) ↓
WSMCDD-2025
Conferences Published ↓
RBS:RH-COVID-19 (2023)
ICMRS'23
PIPRDA-2023
Contact Us
Plagiarism is checked by the leading plagiarism checker
Call for Paper
Volume 6 Issue 4
July-August 2024
Indexing Partners






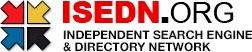

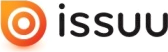


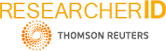
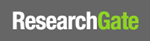
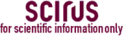
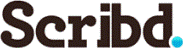

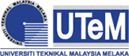
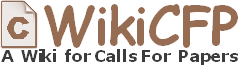
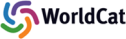
From Data to Impact: Machine Learning Models for Sustainable Development Region Classification- Accelerating the Achievement of Sustainable Development Goals through Predictive Analytics
Author(s) | Deepshika Vijayanand |
---|---|
Country | India |
Abstract | This research paper presents a classification model for predicting the region to which a country belongs based on its sustainability scores and other related features. The dataset used in this study comprises comprehensive data on sustainability and progress towards achieving the Sustainable Development Goals (SDGs) for various countries. The primary objective is to understand regional trends in sustainability and assess countries' progress in sustainable development. The research begins with data preparation and preprocessing steps, including merging datasets, handling missing values, and standardizing features. Exploratory data analysis is performed to visualize the distribution of the target variable (region) and the distributions of numeric features related to SDG scores. Additionally, relationships between these features are explored using correlation matrices and pair plots. Several machine learning models are employed to classify countries into their respective regions. The models used include Random Forest, Support Vector Machine (SVM) with a linear kernel, K-Nearest Neighbors (KNN), Logistic Regression, Decision Tree, and SVM with a radial basis function (RBF) kernel. Each model is trained on the dataset, and their performance is evaluated in terms of accuracy, precision, recall, and F1-score. The results demonstrate the effectiveness of these models in accurately classifying countries into regions based on sustainability scores and other attributes. The findings reveal that Random Forest, K-Nearest Neighbors, Decision Tree, and SVM with RBF kernel achieve exceptionally high accuracy, suggesting their suitability for regional classification based on sustainability metrics. Logistic Regression and SVM with a linear kernel also provide competitive results. In conclusion, this research contributes to understanding regional trends in sustainability by utilizing machine learning models to predict the regions of countries based on their sustainability scores and associated features. Such predictive models can be valuable tools for policymakers and organizations seeking to assess and address regional disparities in sustainable development progress. |
Keywords | Sustainable Development Goals (SDGs), Regional Classification, Machine Learning, Data Science, Predictive Modeling. Data-Driven Analysis |
Field | Computer > Data / Information |
Published In | Volume 5, Issue 5, September-October 2023 |
Published On | 2023-10-05 |
Cite This | From Data to Impact: Machine Learning Models for Sustainable Development Region Classification- Accelerating the Achievement of Sustainable Development Goals through Predictive Analytics - Deepshika Vijayanand - IJFMR Volume 5, Issue 5, September-October 2023. DOI 10.36948/ijfmr.2023.v05i05.7180 |
DOI | https://doi.org/10.36948/ijfmr.2023.v05i05.7180 |
Short DOI | https://doi.org/gst3s7 |
Share this
E-ISSN 2582-2160

CrossRef DOI is assigned to each research paper published in our journal.
IJFMR DOI prefix is
10.36948/ijfmr
Downloads
All research papers published on this website are licensed under Creative Commons Attribution-ShareAlike 4.0 International License, and all rights belong to their respective authors/researchers.
