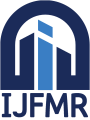
International Journal For Multidisciplinary Research
E-ISSN: 2582-2160
•
Impact Factor: 9.24
A Widely Indexed Open Access Peer Reviewed Multidisciplinary Bi-monthly Scholarly International Journal
Home
Research Paper
Submit Research Paper
Publication Guidelines
Publication Charges
Upload Documents
Track Status / Pay Fees / Download Publication Certi.
Editors & Reviewers
View All
Join as a Reviewer
Reviewer Referral Program
Get Membership Certificate
Current Issue
Publication Archive
Conference
Publishing Conf. with IJFMR
Upcoming Conference(s) ↓
WSMCDD-2025
Conferences Published ↓
RBS:RH-COVID-19 (2023)
ICMRS'23
PIPRDA-2023
Contact Us
Plagiarism is checked by the leading plagiarism checker
Call for Paper
Volume 6 Issue 4
July-August 2024
Indexing Partners






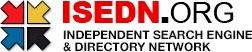

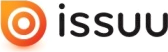


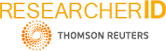
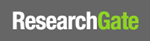
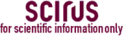
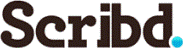

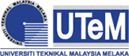
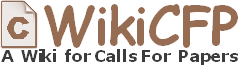
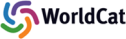
Real-time Card Fraud Detection: A Stacked Ensemble Machine Learning Approach
Author(s) | SATHWIK RAO NADIPELLI, Medha Mittal, Avi Das, Rohit Srinivas Shibineni, Karthik Kumar Reddy Kota |
---|---|
Country | India |
Abstract | As the world witnesses, Card fraud detection has become more and more crucial observes a rise in new and innovative techniques used by offenders. Machine learning and artificial intelligence are at the forefront of the various fraud detection technologies that credit card firms and financial institutions are utilizing. The Nilson Report's startling statistics demonstrate the scope of the issue. Global losses due to credit and debit card fraud increased to $40.6 billion in 2022, up from $34.7 billion the year before, with the United States being responsible for a large 36.8% of these losses. Additionally, younger people, especially those between the ages of 20 and 29, were disproportionately affected by credit card fraud. Our study is unique in a number of respects. We used a carefully controlled dataset to guarantee that the values after preprocessing were accurate and complete. We enhanced the detection of fraudulent transactions by our model by using a wide range of features. To find the most suitable machine learning algorithm for our dataset, we also investigated a range of other techniques. Our study used a dataset with the transaction histories of over 300,000 people from the UCI Machine Learning Repository. We determined the best methods through a multi-step procedure that included data pretreatment, dataset division, and model selection. Our study is unique in a number of respects. We used a carefully controlled dataset to guarantee that the values after preprocessing were accurate and complete. We enhanced the detection of fraudulent transactions by our model by using a wide range of features. To find the most suitable machine learning algorithm for our dataset, we also investigated a range of other techniques. The Stacked Ensemble model, SN Algorithm (SVM + Naive Bayes), beat other models according to the results in terms of important metrics. Impressive AUC, CA, F1, accuracy, and recall ratings demonstrated the system's effectiveness in preventing card theft. |
Keywords | Machine Learning, Card fraud regression, Ensembled algorithm, Data visualization |
Field | Engineering |
Published In | Volume 5, Issue 5, September-October 2023 |
Published On | 2023-10-12 |
Cite This | Real-time Card Fraud Detection: A Stacked Ensemble Machine Learning Approach - SATHWIK RAO NADIPELLI, Medha Mittal, Avi Das, Rohit Srinivas Shibineni, Karthik Kumar Reddy Kota - IJFMR Volume 5, Issue 5, September-October 2023. DOI 10.36948/ijfmr.2023.v05i05.7468 |
DOI | https://doi.org/10.36948/ijfmr.2023.v05i05.7468 |
Short DOI | https://doi.org/gsv6b7 |
Share this
E-ISSN 2582-2160

CrossRef DOI is assigned to each research paper published in our journal.
IJFMR DOI prefix is
10.36948/ijfmr
Downloads
All research papers published on this website are licensed under Creative Commons Attribution-ShareAlike 4.0 International License, and all rights belong to their respective authors/researchers.
