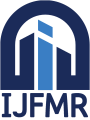
International Journal For Multidisciplinary Research
E-ISSN: 2582-2160
•
Impact Factor: 9.24
A Widely Indexed Open Access Peer Reviewed Multidisciplinary Bi-monthly Scholarly International Journal
Home
Research Paper
Submit Research Paper
Publication Guidelines
Publication Charges
Upload Documents
Track Status / Pay Fees / Download Publication Certi.
Editors & Reviewers
View All
Join as a Reviewer
Reviewer Referral Program
Get Membership Certificate
Current Issue
Publication Archive
Conference
Publishing Conf. with IJFMR
Upcoming Conference(s) ↓
WSMCDD-2025
Conferences Published ↓
RBS:RH-COVID-19 (2023)
ICMRS'23
PIPRDA-2023
Contact Us
Plagiarism is checked by the leading plagiarism checker
Call for Paper
Volume 6 Issue 4
July-August 2024
Indexing Partners






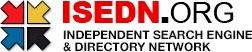

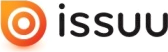


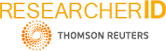
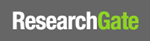
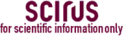
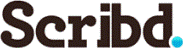

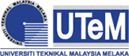
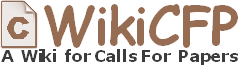
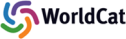
Ensemble Learning for Enhanced Breast Cancer Detection: A Machine Learning Approach
Author(s) | Snigdha Bairi |
---|---|
Country | India |
Abstract | Breast cancer is the most common cancer among women worldwide, with early detection being crucial for improved patient outcomes. Traditional machine learning algorithms have been employed in this domain, but their performance can be limited when dealing with complex medical datasets. This study investigates the potential of ensemble learning to enhance breast cancer detection accuracy. We implemented and evaluated popular ensemble learning methods on a well-established breast cancer dataset. |
Field | Computer > Artificial Intelligence / Simulation / Virtual Reality |
Published In | Volume 5, Issue 5, September-October 2023 |
Published On | 2023-10-19 |
Cite This | Ensemble Learning for Enhanced Breast Cancer Detection: A Machine Learning Approach - Snigdha Bairi - IJFMR Volume 5, Issue 5, September-October 2023. DOI 10.36948/ijfmr.2023.v05i05.7707 |
DOI | https://doi.org/10.36948/ijfmr.2023.v05i05.7707 |
Short DOI | https://doi.org/gswg5x |
Share this
E-ISSN 2582-2160

CrossRef DOI is assigned to each research paper published in our journal.
IJFMR DOI prefix is
10.36948/ijfmr
Downloads
All research papers published on this website are licensed under Creative Commons Attribution-ShareAlike 4.0 International License, and all rights belong to their respective authors/researchers.
