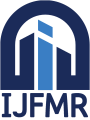
International Journal For Multidisciplinary Research
E-ISSN: 2582-2160
•
Impact Factor: 9.24
A Widely Indexed Open Access Peer Reviewed Multidisciplinary Bi-monthly Scholarly International Journal
Home
Research Paper
Submit Research Paper
Publication Guidelines
Publication Charges
Upload Documents
Track Status / Pay Fees / Download Publication Certi.
Editors & Reviewers
View All
Join as a Reviewer
Get Membership Certificate
Current Issue
Publication Archive
Conference
Publishing Conf. with IJFMR
Upcoming Conference(s) ↓
WSMCDD-2025
GSMCDD-2025
AIMAR-2025
Conferences Published ↓
ICCE (2025)
RBS:RH-COVID-19 (2023)
ICMRS'23
PIPRDA-2023
Contact Us
Plagiarism is checked by the leading plagiarism checker
Call for Paper
Volume 7 Issue 4
July-August 2025
Indexing Partners






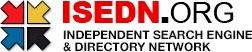

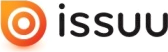


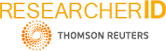
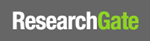
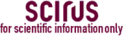
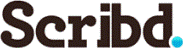

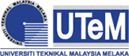
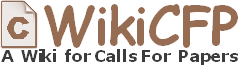
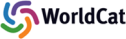
Predictive Modeling of Chronic Kidney Disease: An Ensemble ML Approach
Author(s) | AVI DAS, SRINIJA PRAVALLIKA PURANAM, HARI VENKATA RAVI TEJA ANUMUKO, GREESHMA GEETHIKA RAMPAM, KOTESWARARAO CH |
---|---|
Country | INDIA |
Abstract | Globally, Chronic kidney disease (CKD) is becoming a significant threat to public health, As Effective management and treatment of CKD depend heavily on early detection. In this study, we propose an in depth approach for CKD detection through stacking of machine learning. We utilized a hospital dataset with 25 features to develop prediction models for the classification of chronic kidney disease. The dataset is intended for a classification challenge and contains multivariate data. After that, the data was divided into training and testing sets using an 80-20 split, making it possible to assess the performance of the model. Many machine learning models were used, however one stacked model that included the Random Forest Classifier, Gradient Boosting Machine (RG), Convolutional Neural Network (CNN), and Decision Tree received special attention. The suitability of these models for the CKD classification assignment led to their selection. After hyperparameter tuning was done to maximize the models' performance, the models were assessed using metrics including AUC, accuracy, F1 score, precision, and recall on the testing dataset. Our research showed that the layered RG model produced very good outcomes. Later, hyperparameter tuning was done to maximize the models' performance, the models were assessed using metrics including AUC, accuracy, F1 score, precision, and recall on the testing dataset. Our results showed that the layered RG model produced very good outcomes. These results demonstrate how machine learning can be used to diagnose chronic kidney disease (CKD) early and have positive effects on healthcare by providing a way to improve patient outcomes and healthcare management. |
Keywords | Feature Engineering, CKD, Ensembled Machine Learning, Hyperparameter Tuning, Stacked Model |
Field | Computer > Artificial Intelligence / Simulation / Virtual Reality |
Published In | Volume 5, Issue 6, November-December 2023 |
Published On | 2023-11-22 |
DOI | https://doi.org/10.36948/ijfmr.2023.v05i06.9264 |
Short DOI | https://doi.org/gs63xj |
Share this

E-ISSN 2582-2160

CrossRef DOI is assigned to each research paper published in our journal.
IJFMR DOI prefix is
10.36948/ijfmr
Downloads
All research papers published on this website are licensed under Creative Commons Attribution-ShareAlike 4.0 International License, and all rights belong to their respective authors/researchers.
