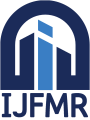
International Journal For Multidisciplinary Research
E-ISSN: 2582-2160
•
Impact Factor: 9.24
A Widely Indexed Open Access Peer Reviewed Multidisciplinary Bi-monthly Scholarly International Journal
Home
Research Paper
Submit Research Paper
Publication Guidelines
Publication Charges
Upload Documents
Track Status / Pay Fees / Download Publication Certi.
Editors & Reviewers
View All
Join as a Reviewer
Get Membership Certificate
Current Issue
Publication Archive
Conference
Publishing Conf. with IJFMR
Upcoming Conference(s) ↓
WSMCDD-2025
GSMCDD-2025
Conferences Published ↓
RBS:RH-COVID-19 (2023)
ICMRS'23
PIPRDA-2023
Contact Us
Plagiarism is checked by the leading plagiarism checker
Call for Paper
Volume 7 Issue 2
March-April 2025
Indexing Partners






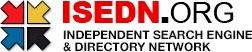

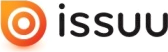


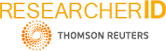
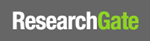
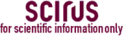
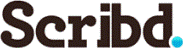

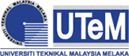
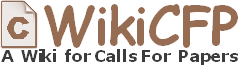
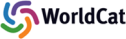
Automated Disease Diagnosis using Deep Learning
Author(s) | Arpit Sarraf, Saikat Sinhamahapatra, Mohit Rana, Amal S, Aman Kumar |
---|---|
Country | India |
Abstract | In this study, a large dataset of medical records, including patient demographics, clinical measurements, and laboratory results, is employed to develop a robust deep learning model. The model utilizes state-of-the-art convolutional neural networks (CNNs) and recurrent neural networks (RNNs) to extract valuable features from multi-modal data sources. These data sources encompass medical images (such as retinal scans and ultrasounds), textual information (patient history, symptoms, and lab reports), and genetic markers. The proposed deep learning model employs both supervised and unsupervised learning techniques. In the supervised phase, the model is trained on labeled data to predict diabetes status accurately. The unsupervised phase leverages the power of deep autoencoders and generative adversarial networks (GANs) to discover latent representations of data, aiding in feature extraction and anomaly detection. The evaluation of the model is conducted on a separate dataset, and its performance is compared to existing diagnostic methods, including traditional clinical assessments and machine learning approaches. The results demonstrate superior accuracy, sensitivity, and specificity in diabetes diagnosis, showcasing the potential of deep learning for improving healthcare outcomes. |
Keywords | Deep learning, Dataset, Machine Learning |
Field | Computer > Data / Information |
Published In | Volume 5, Issue 6, November-December 2023 |
Published On | 2023-11-29 |
DOI | https://doi.org/10.36948/ijfmr.2023.v05i06.9469 |
Short DOI | https://doi.org/gs63th |
Share this

E-ISSN 2582-2160

CrossRef DOI is assigned to each research paper published in our journal.
IJFMR DOI prefix is
10.36948/ijfmr
Downloads
All research papers published on this website are licensed under Creative Commons Attribution-ShareAlike 4.0 International License, and all rights belong to their respective authors/researchers.
