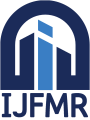
International Journal For Multidisciplinary Research
E-ISSN: 2582-2160
•
Impact Factor: 9.24
A Widely Indexed Open Access Peer Reviewed Multidisciplinary Bi-monthly Scholarly International Journal
Home
Research Paper
Submit Research Paper
Publication Guidelines
Publication Charges
Upload Documents
Track Status / Pay Fees / Download Publication Certi.
Editors & Reviewers
View All
Join as a Reviewer
Reviewer Referral Program
Get Membership Certificate
Current Issue
Publication Archive
Conference
Publishing Conf. with IJFMR
Upcoming Conference(s) ↓
WSMCDD-2025
GSMCDD-2025
Conferences Published ↓
RBS:RH-COVID-19 (2023)
ICMRS'23
PIPRDA-2023
Contact Us
Plagiarism is checked by the leading plagiarism checker
Call for Paper
Volume 6 Issue 6
November-December 2024
Indexing Partners






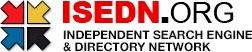

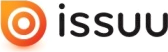


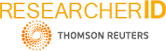
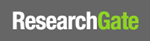
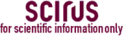
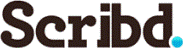

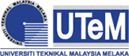
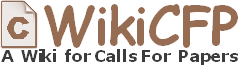
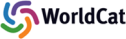
NEUROSYNTH: Enhancing Cognitive Computing using Deep Neural Networks
Author(s) | Himanshu, Shubhrika Sharma, Aakash, Vineet Mehan |
---|---|
Country | India |
Abstract | This study presents an innovative approach to enhance question-answering (QA) systems that utilize a RoBERTa-based architecture and complexity-enhanced input features. The work is divided into four primary parts: training methodology, feature engineering, building models, and data preprocessing. We propose a Python function that uses readability measures and natural language processing techniques to calculate the linguistic difficulty metrics for input sentences. TensorFlow Datasets (TFDS) are then used to load and preprocess the SquAD (Stanford Question Answering Dataset) dataset to enable effective training. Word embeddings from previously trained GloVe vectors are integrated with complexity metrics to prepare input features that add contextual information to the input representation. With the inclusion of the enriched features, a distinctive question-answering model based on the RoBERTa architecture is trained using the AdamW optimizer and CrossEntropyLoss. Iterative epochs are used in the training process to optimize the model's parameters and minimize the loss function. An independent validation dataset is used to evaluate the model's performance, proving the usefulness of the suggested method in improving the accuracy and robustness of the QA system. All in all, this work offers an organized strategy for improving the quality of systems by fusing cutting-edge neural architecture with input properties that are increased by complexity. |
Keywords | NLU, NLP, QA, RoBERTa, Complexity Analysis, Word Embeddings, GloVe, SQuAD |
Field | Computer > Artificial Intelligence / Simulation / Virtual Reality |
Published In | Volume 6, Issue 2, March-April 2024 |
Published On | 2024-04-29 |
Cite This | NEUROSYNTH: Enhancing Cognitive Computing using Deep Neural Networks - Himanshu, Shubhrika Sharma, Aakash, Vineet Mehan - IJFMR Volume 6, Issue 2, March-April 2024. DOI 10.36948/ijfmr.2024.v06i02.18730 |
DOI | https://doi.org/10.36948/ijfmr.2024.v06i02.18730 |
Short DOI | https://doi.org/gtsg4h |
Share this

E-ISSN 2582-2160

CrossRef DOI is assigned to each research paper published in our journal.
IJFMR DOI prefix is
10.36948/ijfmr
Downloads
All research papers published on this website are licensed under Creative Commons Attribution-ShareAlike 4.0 International License, and all rights belong to their respective authors/researchers.
