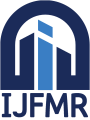
International Journal For Multidisciplinary Research
E-ISSN: 2582-2160
•
Impact Factor: 9.24
A Widely Indexed Open Access Peer Reviewed Multidisciplinary Bi-monthly Scholarly International Journal
Home
Research Paper
Submit Research Paper
Publication Guidelines
Publication Charges
Upload Documents
Track Status / Pay Fees / Download Publication Certi.
Editors & Reviewers
View All
Join as a Reviewer
Reviewer Referral Program
Get Membership Certificate
Current Issue
Publication Archive
Conference
Publishing Conf. with IJFMR
Upcoming Conference(s) ↓
WSMCDD-2025
Conferences Published ↓
RBS:RH-COVID-19 (2023)
ICMRS'23
PIPRDA-2023
Contact Us
Plagiarism is checked by the leading plagiarism checker
Call for Paper
Volume 6 Issue 4
July-August 2024
Indexing Partners






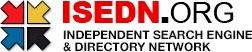

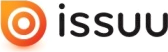


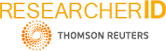
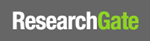
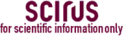
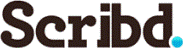

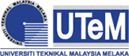
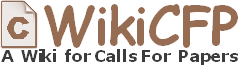
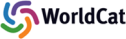
Energy Pooling Market Price Forecasting using Machine Learning
Author(s) | Y.Shanmukha Manohara Reddy, B.Srilatha, Ch.Yaswanth, P.Dilip |
---|---|
Country | India |
Abstract | In competitive electricity markets, accurate price forecasting is required to both power producers and consumers for planning their bidding strategies in order to maximize their own benefits. Price classification is an alternative approach to fore casting where the exact values of future prices are not mandatory. Presently, two efficient algorithms are proposed for both short term price forecasting (STPF) and classification (STPC) purposes. The algorithms include various methodologies like wavelet trans form (WT), fuzzy adaptive particle swarm optimization (FA-PSO) and feed forward neural networks (FFNN). WT is utilized to con vert the pathetic price series to an inviolable price series without losing the originality in the signal. Standard PSO (C) is implemented to tune the fixed architecture FFNN weights and biases. In the present nonlinear problem, linear variation of inertia weight does not resemble exact search process. Hence, dynamic inertia weight is accomplished by implementing the fuzzy systems in the GRADIENTBOOSTINGREGRESSOR approach. The hybrid methodology is implemented on Spanish electricity markets for the year 2002. To validate, three types of price classes and historical price series that are utilized by many researches as input features, are considered. Various statistical indicators are evaluated to compare and validate the proposed approaches with the past approaches available in the literature survey. Index Terms—Forecasting, fuzzy systems, neural networks (NN), particle swarm optimization (PSO), wavelet transform (WT). |
Keywords | gradient boosting regressor,random forest, support vector , root mean square error,indian energy exchange , |
Field | Engineering |
Published In | Volume 6, Issue 3, May-June 2024 |
Published On | 2024-06-21 |
Cite This | Energy Pooling Market Price Forecasting using Machine Learning - Y.Shanmukha Manohara Reddy, B.Srilatha, Ch.Yaswanth, P.Dilip - IJFMR Volume 6, Issue 3, May-June 2024. DOI 10.36948/ijfmr.2024.v06i03.19133 |
DOI | https://doi.org/10.36948/ijfmr.2024.v06i03.19133 |
Short DOI | https://doi.org/gt2cb6 |
Share this
E-ISSN 2582-2160

CrossRef DOI is assigned to each research paper published in our journal.
IJFMR DOI prefix is
10.36948/ijfmr
Downloads
All research papers published on this website are licensed under Creative Commons Attribution-ShareAlike 4.0 International License, and all rights belong to their respective authors/researchers.
