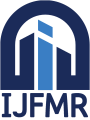
International Journal For Multidisciplinary Research
E-ISSN: 2582-2160
•
Impact Factor: 9.24
A Widely Indexed Open Access Peer Reviewed Multidisciplinary Bi-monthly Scholarly International Journal
Home
Research Paper
Submit Research Paper
Publication Guidelines
Publication Charges
Upload Documents
Track Status / Pay Fees / Download Publication Certi.
Editors & Reviewers
View All
Join as a Reviewer
Get Membership Certificate
Current Issue
Publication Archive
Conference
Publishing Conf. with IJFMR
Upcoming Conference(s) ↓
WSMCDD-2025
GSMCDD-2025
Conferences Published ↓
RBS:RH-COVID-19 (2023)
ICMRS'23
PIPRDA-2023
Contact Us
Plagiarism is checked by the leading plagiarism checker
Call for Paper
Volume 7 Issue 2
March-April 2025
Indexing Partners






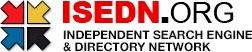

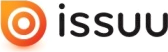


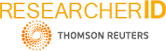
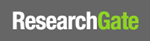
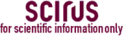
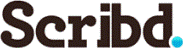

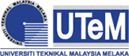
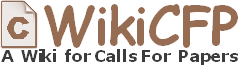
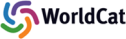
Breast Cancer Detection using CNN
Author(s) | Ranjeet Yadav, Saurabh Maurya, Shivam Sharma, Sumit Gaurav, Mr. Madhup Agrawal |
---|---|
Country | India |
Abstract | Breast cancer stands as a leading cause of cancer- related fatalities worldwide. Assessing cancer accurately through eosin-stained images remains a complex task, often resulting in discrepancies among medical professionals while reaching a con- clusive diagnosis. To streamline this intricate process, Computer- Aided Diagnosis (CAD) systems present a promising avenue, aiming to reduce costs and enhance efficiency. Traditional clas- sification methods hinge on problem-specific feature extraction, rooted in domain knowledge. However, addressing the multitude of challenges posed by these feature-centric techniques has led to the emergence of deep learning methods as viable alternatives. Here, we propose a novel approach employing Convolutional Neural Networks (CNNs) for the classification of hematoxylin and eosin-stained breast biopsy images. Our method categorizes images into four distinct groups: normal tissue, benign lesion, in situ carcinoma, and invasive carcinoma. Additionally, it per- forms a binary classification distinguishing carcinoma from non- carcinoma cases. The meticulously designed network architecture facilitates information extraction across multiple scales, encom- passing both individual nuclei and overall tissue organization. This design choice enables seamless integration of our proposed system with wholeslide histology images. Notably, our method achieves a commendable accuracy of 77.8four-class classification and demonstrates a high sensitivity of 95.6% in identifying cancer cases. |
Keywords | Breast cancer detection, Convolutional neural network (CNN), Mammogram images ,Deep learning, Image recognition ,Benign vs. malignant classification ,Early detection ,Diagnosis, Medical imaging ,Feature extraction. |
Field | Engineering |
Published In | Volume 6, Issue 3, May-June 2024 |
Published On | 2024-05-26 |
DOI | https://doi.org/10.36948/ijfmr.2024.v06i03.21254 |
Short DOI | https://doi.org/gtwmns |
Share this

E-ISSN 2582-2160

CrossRef DOI is assigned to each research paper published in our journal.
IJFMR DOI prefix is
10.36948/ijfmr
Downloads
All research papers published on this website are licensed under Creative Commons Attribution-ShareAlike 4.0 International License, and all rights belong to their respective authors/researchers.
