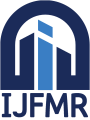
International Journal For Multidisciplinary Research
E-ISSN: 2582-2160
•
Impact Factor: 9.24
A Widely Indexed Open Access Peer Reviewed Multidisciplinary Bi-monthly Scholarly International Journal
Home
Research Paper
Submit Research Paper
Publication Guidelines
Publication Charges
Upload Documents
Track Status / Pay Fees / Download Publication Certi.
Editors & Reviewers
View All
Join as a Reviewer
Get Membership Certificate
Current Issue
Publication Archive
Conference
Publishing Conf. with IJFMR
Upcoming Conference(s) ↓
WSMCDD-2025
GSMCDD-2025
Conferences Published ↓
RBS:RH-COVID-19 (2023)
ICMRS'23
PIPRDA-2023
Contact Us
Plagiarism is checked by the leading plagiarism checker
Call for Paper
Volume 7 Issue 2
March-April 2025
Indexing Partners






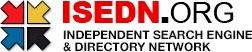

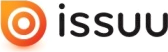


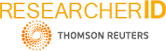
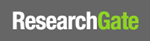
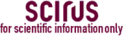
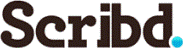

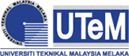
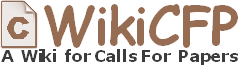
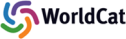
A Review of Evaluating Deep Learning Techniques in Hepatic Cancer Imaging: Automated Segmentation and Tumor Quantification
Author(s) | Yahya Abdul Rehman Shah, Hamza Ahmed Qureshi, Sara Muddassir Qureshi, Saad Ur Rehman Shah, Ashish Shiwlani, Ahsan Ahmad |
---|---|
Country | Pakistan |
Abstract | The classification and treatment of liver cancer, especially hepatocellular carcinoma (HCC), has been one of the significant problems in diagnosing and treating the disease. Previously, segmentation was done through manual processes, which were labour-intensive and highly variable. As a result, new methods for deep learning have been introduced. This article reviews the literature on using deep learning models for the automated segmentation of liver tumours using images focusing on their performance. The constraint for this review paper will be whether deep learning models improve diagnosis and treatment in people with hepatic cancer. In conformity with the PRISMA stipulations, 1295 distinct studies from 2014 to 2024 were examined, of which 100 were included in the final review. The investigation was conducted on various deep learning models’ performance measures to aid segmentation accuracy for U-Net and DeepLabv3+. Despite the difficulties, such as having diverse qualities of the data set, the generalizability of models themselves and the effects of observer variability on ground truth markings severely impact reported performance measures. Therefore, these two architectures are the most common among others and provide good accuracy, with the mean Dices index being 0.87 and 0.89, respectively. When it comes to the automatic segmentation of liver tumours, deep learning models, especially U-Net and DeepLabv3+, seem very promising due to their high precision in identifying target organs or structures within an image. Yet researchers still need to focus more on issues related to dataset variation, standardisation of evaluation metrics and their adoption in clinical settings. For instance, comprehensive validation should be conducted across different patient populations and imaging modalities to guarantee that it can be used in practice; otherwise, one may only have theory without application. |
Keywords | Hepatic cancer, Deep learning, Tumor burden, Automated segmentation and Quantification, Convolutional Neural Networks (CNNs), U-Net, DeepLabv3+. |
Field | Computer > Artificial Intelligence / Simulation / Virtual Reality |
Published In | Volume 6, Issue 5, September-October 2024 |
Published On | 2024-09-12 |
DOI | https://doi.org/10.36948/ijfmr.2024.v06i05.26719 |
Short DOI | https://doi.org/gwfgzm |
Share this

E-ISSN 2582-2160

CrossRef DOI is assigned to each research paper published in our journal.
IJFMR DOI prefix is
10.36948/ijfmr
Downloads
All research papers published on this website are licensed under Creative Commons Attribution-ShareAlike 4.0 International License, and all rights belong to their respective authors/researchers.
