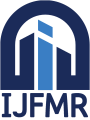
International Journal For Multidisciplinary Research
E-ISSN: 2582-2160
•
Impact Factor: 9.24
A Widely Indexed Open Access Peer Reviewed Multidisciplinary Bi-monthly Scholarly International Journal
Home
Research Paper
Submit Research Paper
Publication Guidelines
Publication Charges
Upload Documents
Track Status / Pay Fees / Download Publication Certi.
Editors & Reviewers
View All
Join as a Reviewer
Get Membership Certificate
Current Issue
Publication Archive
Conference
Publishing Conf. with IJFMR
Upcoming Conference(s) ↓
WSMCDD-2025
GSMCDD-2025
Conferences Published ↓
RBS:RH-COVID-19 (2023)
ICMRS'23
PIPRDA-2023
Contact Us
Plagiarism is checked by the leading plagiarism checker
Call for Paper
Volume 7 Issue 2
March-April 2025
Indexing Partners






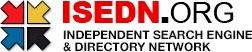

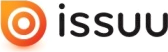


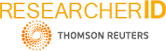
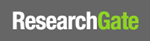
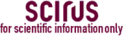
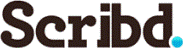

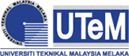
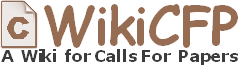
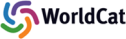
Compqual-Tgnet: A Novel Hybrid Temporal-Graph Neural Architecture for Analyzing Competency and Quality Metrics in Oil and Gas Operations
Author(s) | Shashank Sawant |
---|---|
Country | United States |
Abstract | This research launches a novel Competency-Quality Temporal-Graph Network (CompQual-TGNet) which serves as a hybrid deep learning (DL) framework to study oil and gas business operations through competency metrics and quality indicators. The system combines Graph Neural Network (GNN) and Temporal Convolutional Network (TCN) technology to analyze multiple operational datasets and make accurate future performance estimates. The preprocessing system builds strong data connections by standardizing values with Z-score normalization while handling missing values via Multivariate Imputation by Chained Equations (MICE), finding outliers through Interquartile Range (IQR) and matching temporal data patterns across different inputs. The system also enhances outputs with advanced feature engineering methods including feature extraction and fusion. The proposed framework uses Multi-View Matrix Factorization (MVMF) to extract shared latent features, and Cross-Dataset Attention Mechanism (CDAM) to identify correlations. Moreover, Dynamic Feature Importance Network (DFIN) to learn optimal feature combinations across datasets, and Cross-Attention Fusion Layer to create context-aware feature representations are employed for fusion. Besides, this development brings together GNN for mapping interdependencies among operational components and personnel competencies and TCN for monitoring operational history and long-term temporal patterns. The adaptive fusion layer uses weighting and attention modules to unite these models to make clear predictions based on domain-relevant predictions. The proposed framework shows improvement through updating graphs during operations, adjusting loss functions to match target requirements, and transferring learned models for constant updating. Using these data sources, the developed CompQual-TGNet predicts operating efficiency and detects quality problems with 94.2% accuracy and outran existing systems. |
Field | Engineering |
Published In | Volume 7, Issue 1, January-February 2025 |
Published On | 2025-02-07 |
DOI | https://doi.org/10.36948/ijfmr.2025.v07i01.36610 |
Short DOI | https://doi.org/g84fbd |
Share this

E-ISSN 2582-2160

CrossRef DOI is assigned to each research paper published in our journal.
IJFMR DOI prefix is
10.36948/ijfmr
Downloads
All research papers published on this website are licensed under Creative Commons Attribution-ShareAlike 4.0 International License, and all rights belong to their respective authors/researchers.
