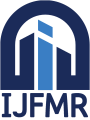
International Journal For Multidisciplinary Research
E-ISSN: 2582-2160
•
Impact Factor: 9.24
A Widely Indexed Open Access Peer Reviewed Multidisciplinary Bi-monthly Scholarly International Journal
Home
Research Paper
Submit Research Paper
Publication Guidelines
Publication Charges
Upload Documents
Track Status / Pay Fees / Download Publication Certi.
Editors & Reviewers
View All
Join as a Reviewer
Get Membership Certificate
Current Issue
Publication Archive
Conference
Publishing Conf. with IJFMR
Upcoming Conference(s) ↓
WSMCDD-2025
GSMCDD-2025
Conferences Published ↓
RBS:RH-COVID-19 (2023)
ICMRS'23
PIPRDA-2023
Contact Us
Plagiarism is checked by the leading plagiarism checker
Call for Paper
Volume 7 Issue 2
March-April 2025
Indexing Partners






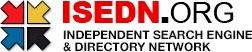

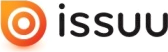


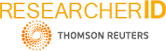
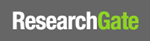
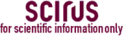
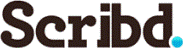

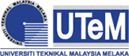
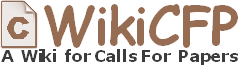
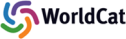
A Review – BearMath: Bearing Fault Diagnostics Using Machine Learning
Author(s) | Mr. Ishan Chandra Joshi, Aayushi Chaudhary, Arjun Thakur |
---|---|
Country | India |
Abstract | Condition monitoring, predictive maintenance, and intelligent fault diagnosis are important for the reliability of rotating machinery and industrial systems. Traditional fault detection methods have been greatly enriched by the recent development of deep learning and advanced signal processing techniques, which harness powerful reactionists, such as CNNs, recurrent architectures, and transfer learning for fail-safe and adaptive fault identification. This review provides a systematic survey of this transition from classical machine condition monitoring approaches (like wavelet transforms and spectral analysis) to modern data-driven deep learning schemes. Drawing on an extensive array of methodologies, such as convolutional and generative adversarial networks (GANs), domain adaptation, and hybrid models that combine deep learning with time frequency representations for enhanced accuracy and generalization, we do a deep dive into the various methods of approach. Emphasis is placed on bearing fault detection, a crucial theme of rotating machinery health monitoring, encompassing a review of the Case Western Reserve University (CWRU) bearing dataset and further benchmark datasets for training and validation. Lastly, we present the challenges/gaps and future research directions, calling for the need for more generalized, interpretable, real-world applicable fault diagnosis models. |
Keywords | Condition-based maintenance, fault diagnosis, deep learning, rotating machinery, signal processing. |
Field | Engineering |
Published In | Volume 7, Issue 2, March-April 2025 |
Published On | 2025-03-25 |
DOI | https://doi.org/10.36948/ijfmr.2025.v07i02.39641 |
Short DOI | https://doi.org/g89v68 |
Share this

E-ISSN 2582-2160

CrossRef DOI is assigned to each research paper published in our journal.
IJFMR DOI prefix is
10.36948/ijfmr
Downloads
All research papers published on this website are licensed under Creative Commons Attribution-ShareAlike 4.0 International License, and all rights belong to their respective authors/researchers.
